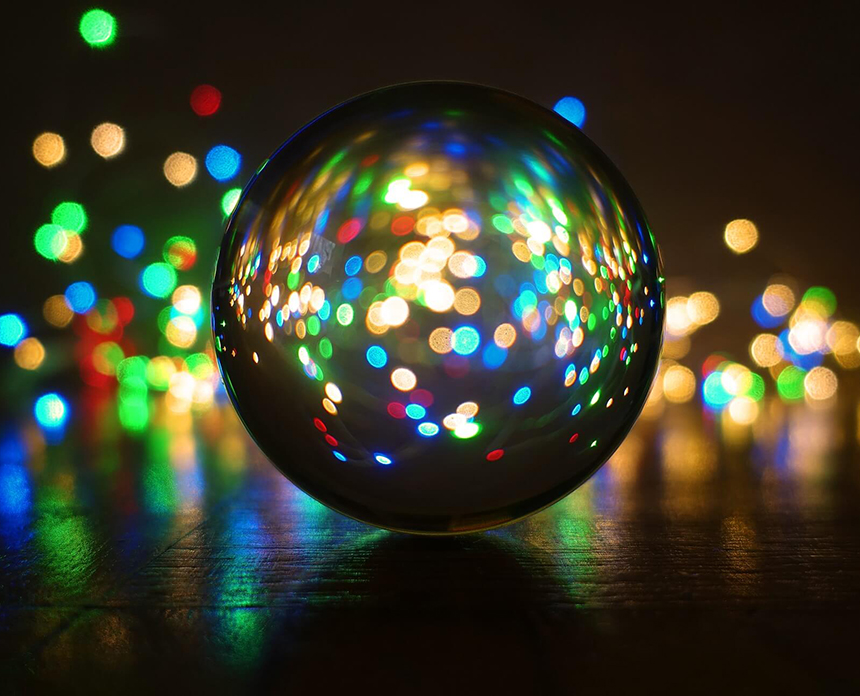
I predict your predictive scoring project will fail. Here’s why and how to do It right
I Predict Your Predictive Scoring Project Will Fail, Here’s Why and How to Do It Right
Predictive scoring was all the rage in 2016, before Account Based Marketing (ABM) became the new, new rage. The buzz around predictive scoring has died down drastically, and there’s a good number of companies that have gone through a full life cycle with this product category, including many of Openprise’s customers and prospects. There seem to be very few long-term success stories with predictive scoring. One of the reasons being that most marketers simply rely on predictive technology as a lazy shortcut to data quality. This assertion may sound strange, but hear me out.
Data Quality Is a Requirement for Predictive Analytics
One of the mantras often repeated at Openprise is, “Garbage-in, garbage-out.” Any process that’s data-driven requires good data as input, and predictive analytics is no exception. Any data scientist will tell you that 80% of their work is data cleansing and preparation. Only 20% of the work is spent on modeling and visualization. So if your sales automation and marketing automation databases have poor quality, how can predictive lead scoring work? One of two ways:
- The initial and periodic manual cleansing is done with professional services.
- The vendor’s product does generic cleansing in the background.
Many predictive analytics technologies, predictive scoring included, require a professional services engagement at the start to clean your database. This ensures that the product has good input data so that the predictions will be good. However, data decays at roughly 25% a year, and at a much faster rate in hot industries with rapidly growing companies. If this manual cleanup effort isn’t kept up at least quarterly, the data quality will quickly deteriorate, and so will the quality of the predictions. Quarterly manual data cleansing is, of course, very expensive and exhausting.
Some vendors handle this data cleansing as embedded functionality in their product. These solutions take one of two approaches:
- Your data is cleaned transparently as part of a “black box” solution and your database isn’t updated with the clean data.
- Your data is matched to, and enriched against, the vendor’s database, which is usually data sourced from a number of data providers like Dun & Bradstreet, DiscoverOrg, or TechTarget.
The first approach is obviously not as good because you don’t get the results of the cleansing. The second approach is more expensive because the data enrichment cost is built-in and often sold as a required second product.
Whatever the approach, data cleansing is a required step, so it’s simply a question of who’s doing it and how it’s done.
Predictive Scoring Should Not be a Shortcut to Data Quality
Almost all the customers and prospects we’ve talked to adopted predictive scoring models before they launched a data quality program. Without a high-quality database that’s well segmented along dimensions such as job function, job level, buyer persona, company size, and industry, it’s impossible to implement a good demographic scoring model. So these customers had two choices, circa 2016:
- Implement a data quality program, then implement scoring, or
- Use a predictive solution that promised to work directly with your raw, dirty databases.
For marketers who are strapped for time and resources with data skills, offloading this problem to a predictive solution was a very attractive shortcut, and many companies took that route. After all, a “predictive” initiative sounds sexier than a “data quality” one. When data quality wasn’t kept up, the performance and value of the predictive score deteriorated quickly.
Whether you’re contemplating adopting predictive scoring or a “recovering user” thinking about giving predictive scoring another chance, here are the important things to consider that can save you the agony of a failed project.
First things first.
Is Predictive Scoring Right For You?
Predictive lead scoring isn’t the right technology for many companies. To help you determine if you are a good fit for the technology, consider the following factors:
Do You Have a Big Enough Database?
Machine learning requires training data, lots of training data. This is another case of garbage-in, garbage-out. In the last few years, even the big tech giants had embarrassing issues with their voice assistant and facial recognition technologies that were traced back to poor training data. Voice assistant, facial recognition, and self-driving cars are examples of machine learning problems that have access to lots of training data that’s generic and not company specific. The challenge with applying machine learning in the B2B context, especially when the data in question is transaction data like opportunities and engagements, is that the database is generally too small to generate good training, and the data is very much company specific, so a vendor can’t leverage learning across their customers’ databases. If you have less than 10,000 opportunities and 10 million engagements, your database is likely too small to generate a robust predictive scoring model.
Do You Need a Black Box or a White Box Technology?
Most machine learning algorithms are black box algorithms. The machine makes predictions based on the training data and it’s actually not easy or even possible to say exactly why the machine came out with any specific prediction. Most companies we’ve talked to that have tried predictive scoring said the results correlated fairly well to close rate, however, they had two general issues:
- The scores were often obviously correlated with a few of the key segments that the customer already knew were important, like job level, company size, and industry. This leads to the question of what exactly was the machine algorithm telling them that they didn’t already know?
- In the cases where the score was not obvious, customers couldn’t figure out how to act on it. One of our customer’s CMO said it well, “I can’t market to a black box.” What he meant was that, while it’s well and good that he knew a lead had a high score, his marketing team still had to create the right content and run the right campaign to nurture the lead. If they didn’t know why a lead scored highly, how could he go about improving his nurturing and targeting strategy? So, black box predictions can be hard to act on, except to tell your sales team that a lead is hot, but you’re not sure why.
A number of predictive solutions say they’re white box solutions. With very few exceptions, then this is not truly machine learning. It’s really just glorified search against a database based on your own Ideal Customer Profile (ICP). So most white box technologies are really just static scoring models wearing a shiny marketing label.
Are Predictive Models Better than Static (White Box) Models?
Most companies have a decent idea about what their ideal customer looks like. Even if they don’t know for sure, with enough historical data in their CRM, they can easily produce that profile based on a segmentation analysis. Companies can’t do this analysis or develop a static demographic scoring model, usually because their database quality is bad and segmentation is even worse. For companies that have invested in improving their database quality and segmentation, then the question becomes, “Does a predictive model really work better than a static (white box) model?” Technology hype and shiny toy syndrome aside, a static model based on a high-quality database has many benefits that should not be overlooked:
- The model tends to be simple and explainable, which makes it possible to do deeper analysis and A/B testing.
- Sales teams will trust a scoring model they understand. Most sales reps have a good ICP in their own head. If marketing’s score doesn’t match that ICP, they’re likely to ignore that lead, regardless of how high marketing says the score is. If the scoring model is transparent and can be understood by sales, it’s more likely they will trust the score.
- Many ICPs are based on segmentation that is very unique to a business. For example, the number of connected devices, number of open job requisitions, size of vehicle fleet. These key segmentations require investment in your database quality. There is no lazy shortcut to incorporate these data into a scoring model.
Final Recommendations
Now you know what it takes to be successful with predictive scoring and know what questions to ask, this is how we recommend you approach the adoption of predictive scoring technology:
- Invest in your database quality and segmentation first. It’s required for all your cool marketing and sales initiatives beyond just scoring.
- Once you have a high-quality database, start with a simple static scoring model and collaborate with your sales team to tune it so they’ll trust it.
- If you’d like to leverage machine learning and predictive analytics, evaluate the solution by benchmarking against your own simple static model. Now you have a concrete way of evaluating the effectiveness of any vendor solution.
Take these steps, and I predict your predictive scoring project will be much more successful.
Recommended resources
Leave a comment