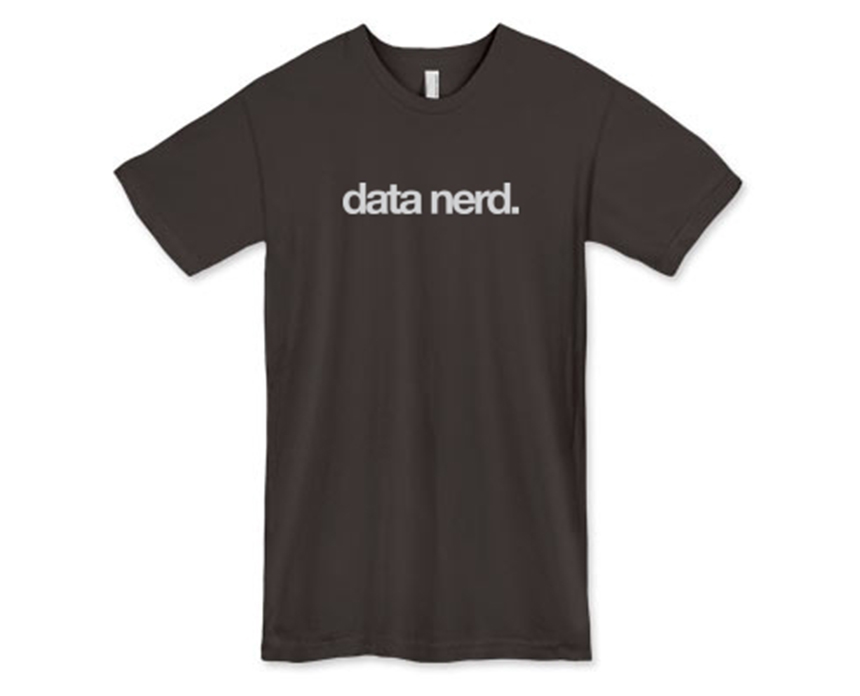
4 critical capabilities of a data-driven marketer
This blog was originally published on Business2Community on October 24, 2015:
https://www.business2community.com/marketing-automation/4-critical-capabilities-data-driven-marketer-01355199#kuBSaus4kQPjCRWs.97
Marketing is going through a paradigm shift. What was once a creative discipline is now blending with hard data science. By using advanced Web and mobile technologies, a business can collect and analyze every interaction it has with its customers and prospects. As these technologies evolve, successful marketers will be those who can extract insight from this vast amount of data and use it to drive new engagement models.
Turning Data into Insights
Data is readily available from Customer Relationship Management (CRM), Marketing Automation Platform (MAP), and various digital channels. Turning data into insights and actions involves three steps: (1) data collection, (2) data processing, and (3) data analysis.
The challenge in turning data into insights is not the lack of, or collection of data. If you know what to measure and what questions to ask, the analysis can easily be delivered by many excellent solutions. The real challenge is the processing step: how to digest this large amount of raw data into a usable form. If you want meaningful insights, you must master these four critical data processing capabilities: data cleansing, normalization, segmentation, extraction, and correlation. Let’s examine these capabilities in more detail.
Cleansing and Normalization
Dirty data is the result of a combination of incomplete data, typos, and incompatible formats. However, even clean data is not necessarily usable data because some data can have too many values. For instance, most applications will interpret the following three account records as three distinct companies:
- Toyota Motors Sales, CA, USA
- Toyota Motor USA, California, U.S.A.
- Toyota Motors Inc., Calif., United States
Normalization is the task of converting variations into a standard value, such as changing “CA”, “Calif.”, and “Cal.” to the standard value “California”.
Data cleansing and normalization makes up the most basic “data hygiene” solution, a tool that should be in every marketer’s kit bag. Look for a solution that is:
- A rule-based solution with highly flexible transformation capabilities that doesn’t require IT support.
- A full set of universal reference data, such as a list of countries, states, and ZIP codes. High-quality reference data greatly simplifies and scales cleansing and normalization tasks. Don’t reinvent the wheel!
- Fuzzy search makes it possible to not anticipate every possible dirty data scenario. Without it, rule maintenance quickly becomes impractical.
Segmentation
Cleaned and normalized data gives you error-free reporting and process automation. However, the new marketing standard practice of targeted campaigns, personalization, and account-based marketing all require advanced segmentation.
The two most widely used segmentations are job title and company size and industry:
Job Title Segmentation
You can often derive job function and job level from a job title. For example, “Director of Network Security” is a manager level person in IT.
To perform job title segmentation, you need a rules engine plus a set of reference data that contains job title keywords and their mapping to job levels and job functions. There are many intricacies involved in what is required of this reference data to achieve a high degree of accuracy. Therefore, when segmenting data it is best to look for a vendor or an agency that can provide the base reference data and offer assistance on how to fine-tune the reference data for your business.
Company Size and Industry Segmentation
Company data such as revenue, the number of employees, and industry is readily available from data services like Dunn & Bradstreet. The challenge is how to translate that third-party data into your specific segmentations. For example:
- A $10M annual revenue company is an “SME” customer for SAP, but a “Large Enterprise” customer for Intuit.
- Is Johnson & Johnson in the healthcare, pharmaceutical, consumer products, or manufacturing industry? The answer depends on what you sell.
Segmentation can take some time to refine, and it needs to evolve with your business, so look for a solution that lets you manage and tweak your segmentation rules without complex reconfiguration.
Extraction
After you build up the basic capabilities of cleaning, normalization, and segmentation, you are ready to discover the hidden treasures of unstructured data. Unstructured data is data such as memos, notes, email threads, chat scripts, and document attachments. Unstructured data contains valuable insights, but only, if you have the means to systematically extract those needles from the haystack.
One powerful example is competitive intelligence. Most B2B companies attempt to collect competitive data about each deal from the sales team, usually with unreliable results. However, this information is often readily available inside notes and memos attached to opportunity records.
So, what options do data marketers have to address these issues? The most effective approach is to look for a rules engine with full-text search capabilities. Traditional database-centric solutions are too slow for this type of application.
Correlation
When working with a marketing technology stack that has many components and databases, extracting insights will require correlating data from multiple sources.
For example, it is useful to know whether a lead in the MAP is from an existing account in the CRM or a net-new lead? This knowledge streamlines lead routing and enables targeted engagements. It requires correlating the MAP’s lead data with the CRM’s account and contact data.
A good data correlation solution should make it simple to aggregate and create relationships among data sets from different sources. Since correlation requires cleansing, normalization, and even segmentation, ideally you should look for a data automation platform that contains all four key capabilities in one easy-to-use solution.
Data-Driven Marketing Starts with Data
Data processing is a fundamental and differentiating capability every data-driven marketer and organization should master. After all, good data is what fuels meaningful reports, predictive analytics, and effective personalization. Simply put, data-driven marketing is impossible without good data. Want inspirations on how to tackle these challenges? Read the 2015 Data Hygiene and Enrichment Report for 14 pages of insights and best practices from SiriusDecisions and other leading B2B companies.
Leave a comment