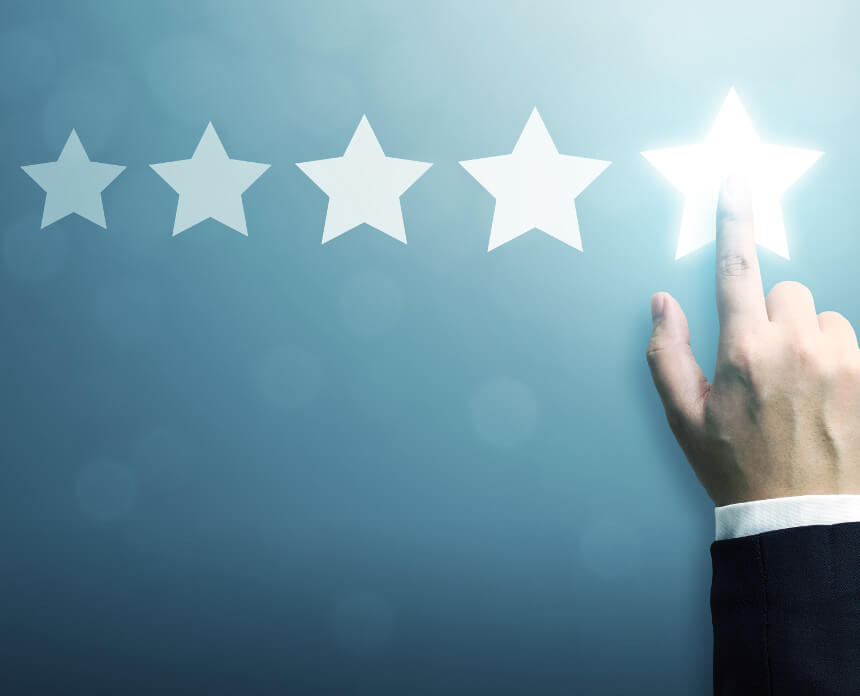
What exactly is data quality for marketing and sales?
It seems like every company we talk to these days has a RevOps data quality initiative going on. Maybe after years of tinkering with the latest and greatest marketing and sales technologies, people have finally figured out there’s no silver bullet or Easy ButtonTM that can give you more leads and higher conversion without doing the hard work of whipping your data into shape. Given that Openprise created the RevOps Automation product category and has been preaching the importance of data, we’re happy to see sales and marketing folks now rightfully focused on data quality. So that begs the question, what exactly is “data quality” for marketing and sales? If you ask ten people to define data quality, you’ll likely get 11 different answers. In almost all cases, the conventional definition of data quality is way, way, way too narrow.
What’s our definition of data quality? We’re glad you asked.
Wikipedia’s definition of data quality is pretty academic. But for marketing and sales professionals, it’s not particularly useful. So let’s define data quality in a more relevant context for sales and marketing professionals.
Data Quality Basics
When most people try to define data quality, they end up with some version of the following attributes. Most of these should be self-explanatory, so we won’t waste your time giving you a bunch of dictionary definitions. Please refer to Wikipedia if you need a refresher:
- Accuracy
- Completeness
- Timeliness
- Format
- Consistency
- Integrity
- Validity
- Precision
Let’s imagine for the moment your data is absolutely pristine and has all the above attributes at 100%. Pure perfection, right? No! Your data can still be (and most likely will be) completely useless. True marketing and sales data quality is data that’s useful to the business, not data that meets some academic criteria.
The key is expanding your view of data quality, so the data you oversee is truly useful to the business.
Usability
No one pursues data quality for fun—not even people at Openprise. 🙂 Data quality’s end goal is either to (1) derive business intelligence or (2) automate business process.
To serve either goal, the data must have good usability. Take the example of industry data. If you have accurate and complete industry data for your entire prospect database—whether it’s SIC, NAICS, or Dun and Bradstreet—you may have over 10,000 different industry values in your data set. Have you ever tried to visualize a data field with 10,000 different values or try to use that data in a business rule? Can you imagine creating a Marketo Smartlist to define a market segment with that many values? It’s downright unusable.
In practice, to make industry data usable, most companies need to map the data down to a list of 15 to 30 industries that are meaningful to the business. This list of 15-30 industries needs to be what is useful to your business, not some list defined by a data provider or industry standard. The industry list that’s most useful to you may be highly “imperfect” from an academic standpoint.
For example, if you sell to specific industries, your industry list may consist of highly granular industries in your area of focus, yet completely broad in areas of non-focus. Say your company sells X-ray equipment. Your industry list is likely to be very granular in healthcare and heavy industries, like hospitals, dental offices, urgent care, gas pipelines, oil exploration, and oil refineries. You’d lump non-focus industries like business services and e-commerce into “Other.”
Other common examples of making data usable include segmenting job title into job function, job level, and buyer persona, or appending metro and urban area data to address.
Relevance
Pop quiz: Is Puerto Rico a country or a state? I know it’s technically a territory, but show me a CRM with a territory field.
The right answer is not what Wikipedia or your third-grade geography teacher says it is—it’s what your sales leadership says it is. If your sales team covers Puerto Rico from the US southeast team, then Puerto Rico should be a state in your CRM. If your sales team covers Puerto Rico from the Latin America team, then Puerto Rico should be a country in your CRM.
Here’s another example. If you’re an Internet of Things technology vendor, you’ll likely have included both Coca-Cola and New York City’s Metropolitan Transportation Authority (MTA) on your target account list, and placed both of these organizations in a custom industry called “vending.” That’s because the MTA’s smart ticket vending machines and Coca-Cola’s Freestyle self-service touch screen soda fountains are relevant for your IoT solution. But any third-party data source will tell you that Coca-Cola’s in the CPG beverage industry and MTA’s in the transportation business. These raw industry data may be accurate, but they’re not relevant for you.
These are just two common examples that show how accurate data may not make the best quality data. Data needs to be interpreted with business context to achieve actual relevancy. And that means relevant data is the best quality data.
Linkage
The most insightful and useful data for your business is data with the necessary linkages to other data, especially other first-party data you own. Here are a few common examples:
- Account-based marketing. You need to know how your prospect data links to the account data in your CRM.
- Customer marketing. You need to be able to differentiate customers vs. prospects and users vs. other people from the same company. That means you better be able to link your marketing database to both customer activity data from your product database and ticketing data from your help desk system.
- Scoring accounts to prioritize sales activity. You need to link your CRM account data with third-party intend data, first-party engagement data across different platforms, and various target account lists.
People aspire to have “360-view of the customer.” But in practice, you don’t always need a “comprehensive” view of the customer to be successful. Depending on your specific goals and purpose, most of the time, you just need to link a few data sets. So while achieving the utopian “360-view of the customer” is great, but can be costly and take too long. So the key is to build data linkages on an as-needed, agile manner.
Final Thoughts on Data Quality
In practice, there’s no single definition of data quality for marketing and sales. Data quality means different things to different companies, departments, projects, processes, and technology stacks. So it’s vital to understand what data quality means to YOU within each context. Data quality is not a generic concept, and it’s meaningless without the proper business context.
So if you’re kicking off a data quality initiative, expand your thinking about what data quality means for your business. Selecting technology to help you with data quality? Think about the capabilities you need to achieve your data quality goals. Is it sufficient to buy some third-party data? Do you need the ability to interpret and segment that data at scale to add usability and relevancy? Do you need the ability to unify and create linkage between all your data sets?
Remember: The best quality data is the most useful data for deriving intelligence and automating business processes. Think bigger than the academic definitions of data quality. Consider these additional dimensions of data quality: usability, relevance, and linkage.
Recommended resources
Leave a comment